Understanding Top-Down and Bottom-Up Ethics in AI Part 1
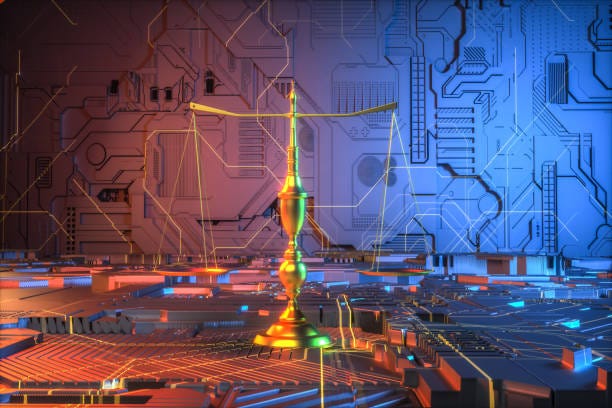
When I was first approaching the subject of top-down and bottom-up ethics in artificial intelligence, I had certain presumptions in mind that began to change as I deepened my research. Originally, I thought these concepts had more to do with ethics either being determined by corporations and governments (coming from the top), or ethics being called for by the people (coming from the bottom).
What I found was that these terms mean something completely different when describing ethics for applied AI. Basically, “top-down” theories amount to rule-utilitarianism and prima-facie deontological ethics, where “bottom-up” refers to case-based reasoning. (van Rysewyk and Pontier 2015) If ethics in AI is programmed based on a system of rules, it is top-down. If ethics in AI is learned from observation, such as machine learning and deep learning, without that base set of rules, it is bottom-up.
Top-down and bottom-up ethics in AI has more to do with how the AI learns ethics. This was enlightening for me. As an anthropologist by training, I am learning the technicalities of how AI is developed. Machines don’t learn as people do. They learn from the data fed to them, and they are very good at certain narrow tasks, such as memorization or data collection, but fall short with things like objective reasoning, which is at the core of ethics. Whether coming from the top-down or bottom-up, the bottom line is that teaching ethics to AI is extremely difficult, both technically and socially.
Many argue that a hybrid of top-down and bottom-up would be the most effective model. Further, some argue that we need to question the ethics of people, both as the producers and consumers of technology, before we can start to assess fairness in AI.
In a paper titled “Artificial Morality: Top-down, Bottom-up, and Hybrid Approaches”, the authors lead by stating: “Artificial morality shifts some of the burden for ethical behavior away from designers and users, and onto the computer systems themselves,” (Allen et. al. 2005)
I would question this. I don’t think that we can hold the machines responsible, ever. We are talking about machines that do not have an inherent conscience or morality as humans do. They don’t work in the same way, and I don’t agree with those that hold them to the same standards. However, AI can act as a mirror, and the problems that arise in AI often reflect the problems we have in society.
AI can act as a mirror, and the problems that arise in AI often reflect the problems we have in society.
We as people need to assume responsibility, both as individuals and as a society at large. Corporations and governments need to cooperate, and individual programmers should continually question and evaluate these systems. In this way, we can use AI technology in an effort to improve society and create a more sustainable world for everyone.
We can look at the Asilomar AI Principles which is a top-down model and has its own critiques. This is an actual list of rules that were put out by the powers that be in tech and AI in hopes to be the guidelines for developing ethics in AI. These things are being thought about and worked on, but they have a long way to go.
We could also look at reinforcement styles of learning, coming from the bottom-up, which is an effective model for teaching people, and see how that is being adopted to teach machines. Reinforcement learning works as a reward or punishment system. Think of the Pavlov experiment, where he trained dogs to drool at the sound of a bell by always ringing a bell before giving them a treat.
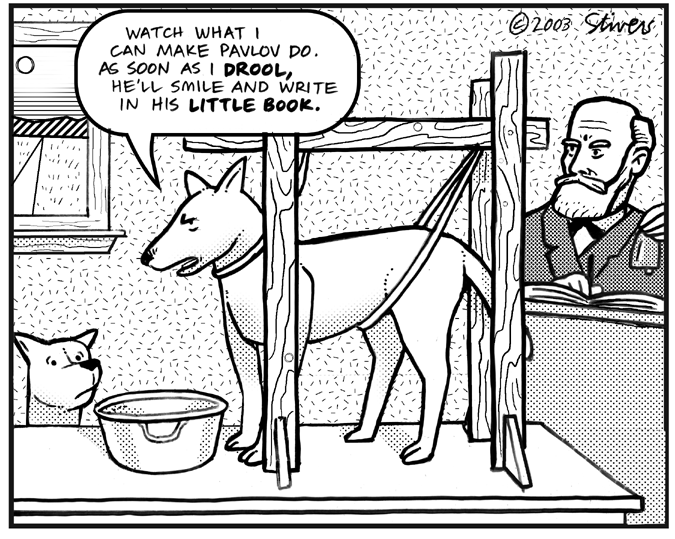
Stivers, M. (2008, December 29).
Pavlov T-shirt now available: Stivers cartoons
. Stivers Cartoons | Cartoons by Mark Stivers, posted every Saturday. Retrieved December 8, 2021, from
https://www.markstivers.com/wordpress/?p=67.
However, some would say it is impossible to teach AI right and wrong if we could even come to an agreement on how to define those terms in the first place. “Although there are shared values that transcend cultural differences, cultures and individuals differ in the details of their ethical systems and mores,” (Wallach et. al, 2005)
The first hard task is to agree on ethics. Look around: We live in a very polarized world. What is fair to some will undoubtedly be unfair to others. Furthermore, “. . . the engineering task of building autonomous systems that safeguard basic human values will force scientists to break down moral decision making into its component parts, recognize what kinds of decisions can and cannot be codified and managed by essentially mechanical systems, and learn how to design cognitive and affective systems capable of managing ambiguity and conflicting perspectives.” (Wallach et. al, 2005)
The Example of Fairness in AI
Many of the common systems of values that experts agree need to be considered in AI include fairness, or to take it further, justice. Let’s work with this concept as an example. We have never had a fair and just world, so to teach an AI to be fair and just doesn’t seem possible. But what if it was? We get into grey areas when we imagine the open-ended potential future of AI, but I would rather imagine it could actually improve the state of things, as opposed to imagining how it could lead to further destruction of humanity.
Artificial intelligence should be fair. The first step is to agree on what a word like fairness means when designing AI. Many people pose the question: Fairness for whom?
Then there is the question of how to teach fairness to AI. AI systems as we know are machines. Machines are good at math. Mathematical fairness and social fairness are two very different things. How can this be codified? Can an equation that solves or tests for fairness between people be developed?
Most AI is built to solve problems of convenience and to automate tedious or monotonous tasks in order to free up our time and make more money. “Computers and robots are largely a product of a materialistic worldview which presupposes a set of metaphysical assumptions that are not always compatible with the spiritual worldviews which produced many of our ethical categories and much of our ethical understanding.” (Allen et. al. 2005)
We see it every day in the algorithms that discriminate and codify what retailers think we are most likely to consume. For example, in the ads they show us, we often see elements that don’t align with our values but rather appeal to our habits of consumption. At the core level, these values are twisted to benefit the current capitalistic systems and have little to do with actually improving our lives. We cannot expect AI to jump from corporate materialism to social justice, or reach a level of fairness, simply by tweaking the algorithms a little.
What I’ve Learned
As with any deep dive into new knowledge, the more I learn, the more I understand just how much I don’t know. I can tell you that teaching ethics to AI is extremely challenging — if not impossible — on multiple fronts. We live in a chaotic and unfair world, so in order to have ethical AI, we need to first evaluate our own ethics. This is the starting place for an equitable AI learning methodology.
We live in a chaotic and unfair world, so in order to have ethical AI, we need to first evaluate our own ethics.
To be continued…
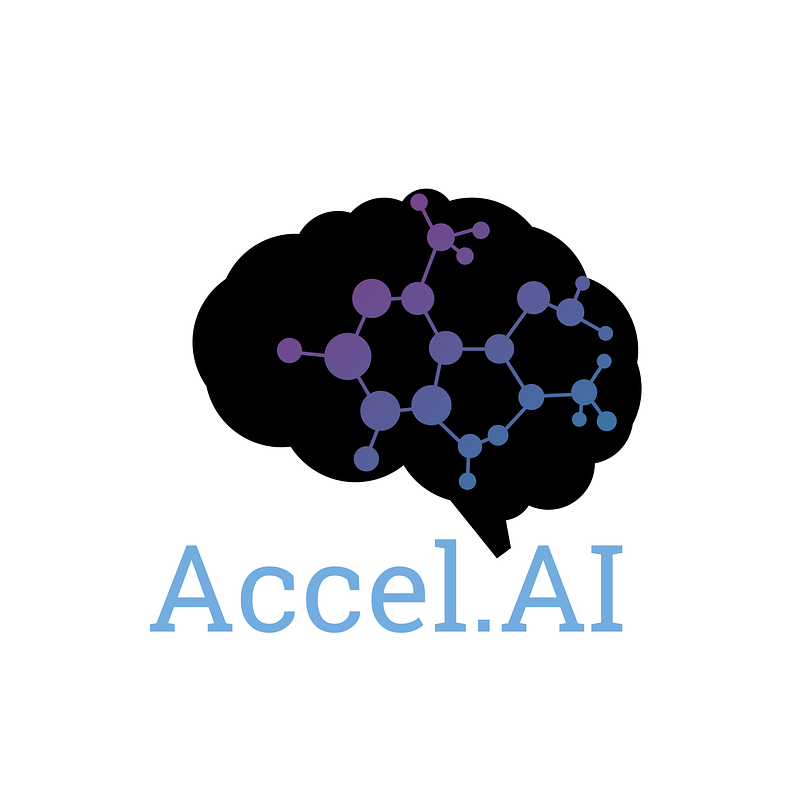
You can stay up to date with Accel.AI; workshops, research, and social impact initiatives through our website, mailing list, meetup group, Twitter, and Facebook.
Join us in driving #AI for #SocialImpact initiatives around the world!
If you enjoyed reading this, you could contribute good vibes (and help more people discover this post and our community) by hitting the 👏 below — it means a lot!
Citations
Artificial morality top-down bottom-up and hybrid approaches. (n.d.). Retrieved December 3, 2021, from https://www.researchgate.net/profile/Wendell-Wallach/publication/225850648_Artificial_Morality_Top-down_Bottom-up_and_Hybrid_Approaches/links/02bfe50d1c8d2c733e000000/Artificial-Morality-Top-down-Bottom-up-and-Hybrid-Approaches.pdf.
Eckart, P. (2020, May 29). Top-down AI: The simpler, data-efficient AI. 10EQS. Retrieved December 3, 2021, from https://www.10eqs.com/knowledge-center/top-down-ai-or-the-simpler-data-efficient-ai/.
Machine morality: Bottom-up and top-down approaches … — AAAI. (n.d.). Retrieved December 3, 2021, from https://www.aaai.org/Papers/Symposia/Fall/2005/FS-05-06/FS05-06-015.pdf.
Stivers, M. (2008, December 29). Pavlov T-shirt now available: Stivers cartoons. Stivers Cartoons | Cartoons by Mark Stivers, posted every Saturday. Retrieved December 8, 2021, from https://www.markstivers.com/wordpress/?p=67.
van Rysewyk, S. P., & Pontier, M. (n.d.). Philarchive/harvest.py at master · GSASTRY/Philarchive. GitHub. Retrieved December 4, 2021, from https://github.com/gsastry/philarchive/blob/master/harvest.py.